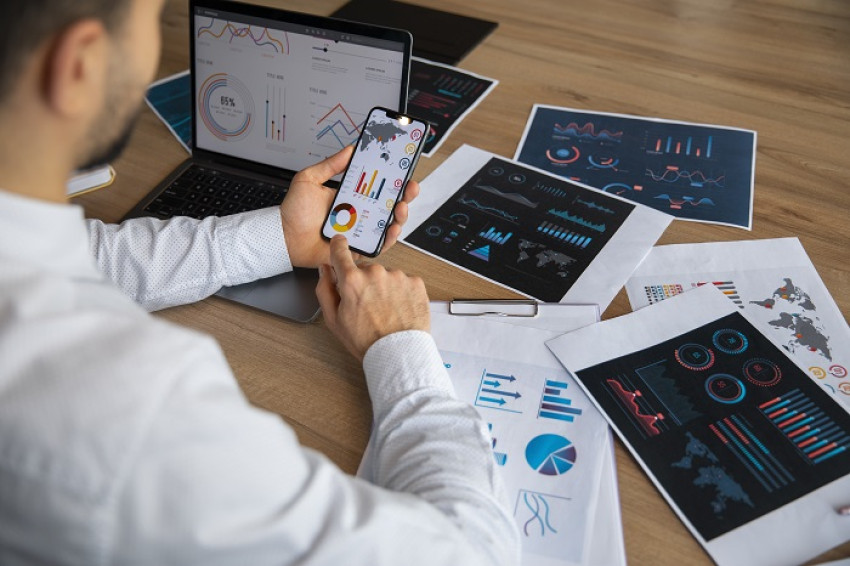
Data science is a complex and multifaceted field that involves a range of skills and techniques to extract insights and knowledge from data. To successfully navigate this field, professionals need to understand the data science life cycle, a systematic approach to solving complex data problems. Enrolling in data science courses or a reputable data science training institute can provide aspiring data scientists with a comprehensive understanding of the data science life cycle and the practical skills needed to succeed in this dynamic field.
In this article, we will explore the data science life cycle in detail, breaking down the key steps and explaining their significance in the data science process. We will cover everything from problem identification and data collection to model building and deployment, providing a comprehensive guide to understanding the data science life cycle. By the end of this article, you will have a solid understanding of the essential steps involved in the data science life cycle and how they work together to solve complex data problems.
What is the Data Science Lifecycle?
The data science lifecycle is a set of processes that data scientists use to solve data problems. It is a structured approach that involves several stages, each of which is designed to help data scientists understand the problem, gather and process the data, create and test models, and finally deploy the solution. The lifecycle is iterative, meaning that data scientists will often cycle through the stages multiple times before arriving at a final solution.
The data science lifecycle has become essential because it provides a structured framework for solving data problems. By following a systematic approach, data scientists can ensure that they are addressing all of the important issues, and not overlooking critical steps in the process.
Steps in the Data Science Lifecycle
There are several steps involved in the data science lifecycle. We will now explain each of these steps in detail.
1. Problem Definition
The first step in the data science lifecycle is to define the problem that needs to be solved. This involves understanding the business problem and identifying the data that will be required to solve it. The problem definition stage is critical because it sets the stage for the entire data science project. If the problem is not well-defined, the project is likely to fail.
2. Data Collection
Once the problem has been defined, the next step is to collect the data that will be required to solve the problem. This involves identifying the sources of data and collecting the data in a usable format. Data collection is often a challenging step because data can come from many different sources, and it is not always clear how to collect and process the data.
3. Data Preparation
Once the data has been collected, the next step is to prepare the data for analysis. This involves cleaning the data, transforming it into a usable format, and selecting the features that will be used in the analysis. Data preparation is a critical step because the quality of the analysis will depend on the quality of the data.
4. Data Analysis
With the data prepared, the next step is to analyze the data. This involves using statistical methods and machine learning algorithms to identify patterns in the data. Data analysis is often an iterative process, where data scientists will experiment with different algorithms and parameters until they find a model that provides accurate results.
5. Model Building
Once the analysis is complete, the next step is to build a model that can be used to solve the problem. This involves selecting an appropriate algorithm and training the model using the data that has been collected. Model building is often an iterative process, where data scientists will experiment with different algorithms and parameters until they find a model that provides accurate results.
6. Model Evaluation
With the model built, the next step is to evaluate its performance. This involves using a test dataset to assess the accuracy of the model. Model evaluation is a critical step because it ensures that the model is accurate and reliable.
7. Model Deployment
Once the model has been evaluated and validated, the final step is to deploy the model. This involves integrating the model into the business process and using it to solve the problem. Model deployment is a critical step because it ensures that the solution is scalable and can be used by the business to make informed decisions.
Summary
In conclusion, understanding the data science life cycle is essential for any aspiring data scientist or professional in the field. By enrolling in a reputable data science training institute or pursuing a data science certification, individuals can gain the practical knowledge and skills needed to excel in this field. The best data science courses provide a comprehensive understanding of the data science life cycle and cover topics such as data collection and preparation, machine learning, statistical analysis, and data visualization.
To succeed in the data science field, individuals need to have a solid understanding of the data science life cycle and the various tools and techniques used to solve complex data problems. Pursuing data scientist training can provide individuals with the foundational knowledge and practical experience needed to excel in this dynamic field. With the right training and certification, individuals can unlock exciting career opportunities in various industries and make a meaningful impact through data-driven insights and solutions.
In a world where data is becoming increasingly valuable, the demand for skilled data scientists is only set to grow. By investing in data science training courses and certification programs, individuals can position themselves at the forefront of this rapidly evolving field and take advantage of the many exciting career opportunities available to them.